Dec 12 2015
Two School of Information Studies (iSchool) faculty members are exploring new ways of combining the efforts of citizen scientists and machine learning algorithms to classify data from a National Science Foundation-funded research initiative called “the most complicated experiment ever undertaken in gravitational physics.”
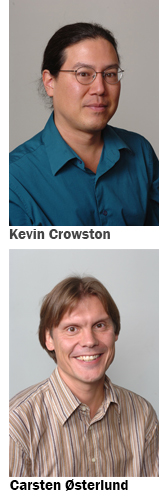
Professor Kevin Crowston and co-investigator Associate Professor Carsten Østerlund are members of a team that has been awarded one million dollars by the National Science Foundation for their project, INSPIRE: Teaming Citizen Science with Machine Learning to Deepen LIGO's View of the Cosmos, (15-47880). The project’s goal is to develop a citizen science system to support the Advanced Laser Interferometer Gravitational Wave Observatory (aLIGO). The aLIGO experiment is expected to open up the window of gravitational wave observations on the Universe before the end of this decade, aLIGO says. The INSPIRE team is headed by principal investigator Vassiliki Kalogera (Northwestern University); and also includes co-principal investigators Joshua Smith (Cal State Fullerton), Shane Larson (Northwestern University) and Laura Trouille (Adler Planetarium).
Drs. Crowston and Østerlund are working to develop a system that combines the strengths of human classification with a machine learning model that learns from the citizen scientists involved in the experiment, while also guiding how information is provided to those participants.
Being able to classify aLIGO data quickly and accurately is important because the high detector sensitivity needed for astrophysical discoveries makes aLIGO very susceptible to noncosmic artifacts and noise—referred to as “glitches”—that must be identified and separated from cosmic signals. While human eyesight is a proven tool for classification, the aLIGO data streams from approximately 30,000 sensors and monitors easily overwhelm a single human. To manage large quantities of data, the project will employ machine-learning techniques, but this approach faces the difficulty of teaching computers to identify and classify the wave artifacts in detector data. Accordingly, the work that Drs. Crowston and Østerlund will contribute aims to maximize the skills both humans and computers can contribute to filtering out non-cosmic incidents, and the interpretations of their findings.
The professors are approaching the development of their system from several vantage points. “Human-centered computing is part of it, but certainly a lot of work is focused on machine learning on the glitches, and the question of how people will interact with those,” Dr. Crowston said. A second focus will be developing learning models that provide a role and educational benefits for volunteers that engage them as partners in scientific discovery as an offset for their labor.
“What we’re trying to do is build in resources for the learner and a trajectory the learner can take so they keep improving their skills,” Dr. Østerlund said. “We want to build in assistance along the way, such as how machine learning can help them sort through glitches, discover at what points they need more information, find out what they need to know about other issues, and how you can assist them in that. “Our angle is, while humans can help the machine to learn, how can the machine learning help the humans to learn.”
Ultimately, their efforts will help aLIGO “quickly identify noise and artifacts in the science data stream, separating out legitimate astrophysical events, and allowing those events to be distributed to other observatories for more detailed source identification and study. This project will also build and evaluate an interface between machine learning and human learning that will itself be an advance on current methods,” the NSF announcement noted.
In addition to this NSF-funded project, Dr. Crowston is the former principal investigator on two others: NSF SOCS Grant 11-11107 ("SOCS: Socially intelligent computing for coding of qualitative data") and, together with Dr. Østerlund, NSF SOCS Grant 12-11071 ("Collaborative Research: Focusing Attention to Improve the Performance of Citizen Science Systems: Beautiful Images and Perceptive Observers.") He and School of Information Studies Professor Jian Qin recently were awarded a research challenge grant from ICPSR for the development and dissemination of a capability maturity model for research data management.
Professor Østerlund is the principal investigator with Professor Steven Sawyer on NSF Grant 12-21945 (“VOSS: Documents and the Doing of Science: Studying Cyberinfrastructures in Use”).
Source: https://ischool.syr.edu/