Quantum mechanics suggests that our universe is fundamentally probabilistic, so how can it be used for something as classical and definitive as computer programming? This article aims to look into the prospects of these two seemingly distinct fields becoming amalgamated to advance knowledge and research in both.
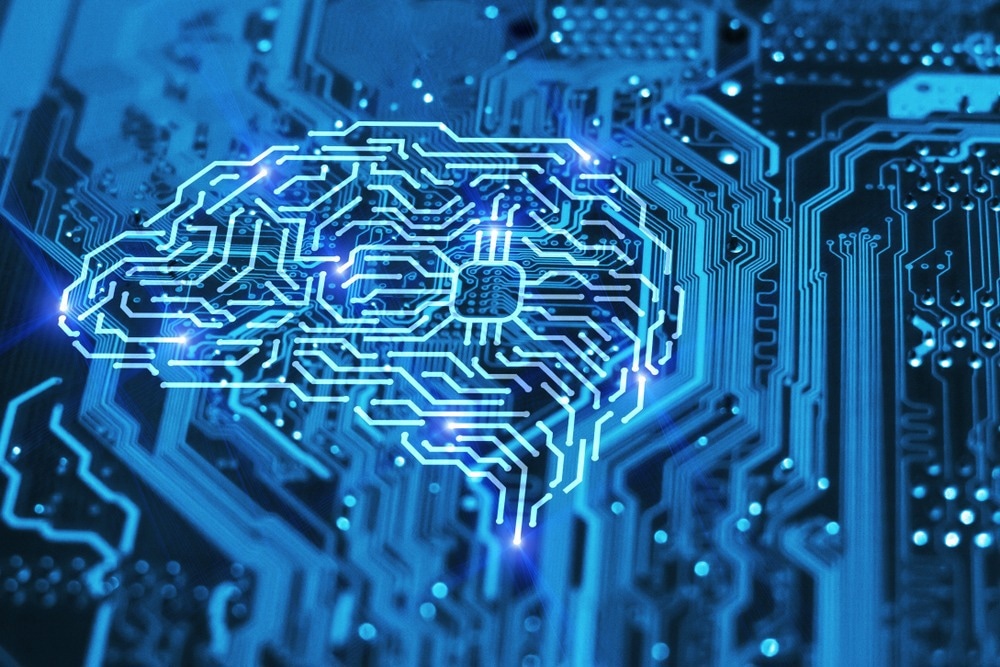
Image Credit: Michael Traitov/Shutterstock.com
Machine learning is a field of computer science that aims to develop computer systems capable of interpreting, analyzing, and drawing conclusions from patterns in data. Machine learning programs have contributed towards face recognition technology and language translation apps and have defeated the world’s best players at classical board games. More recently, many conceptual connections have been found to exist between machine learning and quantum mechanics. Quantum physics is an extremely contemporary topic; only earlier this month, the 2022 Nobel Prize was awarded for experiments entangled photons, establishing the violation of Bell inequalities and pioneering quantum information science.
What can Machine Learning do for Quantum Physics?
A significant field of study in machine learning is creating artificial neural networks. These are computer programs modeled on the human brain. Current models are highly abstract and simplified, but our understanding grows every year. In the last decade, there has been much progress toward creating artificial neural networks that can represent quantum states and solve quantum many-body problems.
One famous example where machine learning is improving our understanding of quantum states is the Ising model. The model states that, below a critical temperature, a magnet is in a ferromagnetic state where all the spin of the atoms in a lattice is ordered. When the temperature is above the critical temperature, the magnet is in a paramagnetic state where the direction of the spins of the atoms is random. Researchers Carrasquilla and Melko used Monte Carlo simulations to conduct machine learning analysis that can efficiently identify whether a magnet is in a ferromagnetic or paramagnetic state. Furthermore, the program could correctly identify the critical temperature for different, unseen lattices.
Artificial neural networks are also extremely adept at representing massively entangled states and show promising signs of solving intricate many-body problems with a large entanglement. The restricted Boltzmann machine (RBM) is a widely used special neural network in the field of machine learning. Researchers Carleo and Troyer introduced a new representation based on this special neural network to study the antiferromagnetic Heisenberg model, and it successfully found both the ground state and time evolution of the model. The new RBM representation can also be applied to studying a certain type of quantum many-body state called a topological state.
What can Quantum Computing do for Machine Learning?
Quantum computing works by exploiting the intrinsic spins of isolated electrons to create a superposition of spin states. They use quantum coherence and entanglement to process information in ways that classical computers can not. Quantum machine learning aims to develop quantum software programs that perform machine learning tasks using quantum information processors.
A quantum speedup is when a quantum algorithm outperforms a classical one. Quantum machine learning can provide exponential speedups over classical computers for a variety of learning tasks. One example of this is a fast Fourier transform, a common classical data analysis technique that is used in machine learning to process large amounts of data. There is a quantum fast Fourier transform counterpart that works exponentially faster than the classical version. This is mathematically described by taking time proportional to log(D) for a D-dimensional vector space, as opposed to taking time proportional to Dlog(D) for classical computers. Another example is searching an unsorted database. For N entries, a quantum computer would take time proportional to √N, whereas a classical computer would take time proportional to N.
Machine learning techniques often involve solving a large number of linear problems, such as matrix multiplication. Quantum mechanics is fundamentally described by linear algebra and therefore has a natural advantage toward solving these problems. In quantum mechanics, scientists manipulate large vectors by decomposing them as tensor networks. One of the best-understood types of tensor networks is the matrix product state, which is being applied to multi-class supervised learning tasks to improve established machine learning programs.
Although highly theoretical at this stage, scientists looking further into the future believe that combining quantum computing encryption analysis with machine learning will boost our understanding of cryptography and will therefore immeasurably improve our understanding of the prime numbers, a grand and important field of study in mathematics. This also plays an important role in the privacy of a user's data.
Conclusion
Both the fields of machine learning and quantum computing are currently in the early development stages, but both will play a pivotal role in the future of 21st-century technology. The potential both fields possess for advancing scientific progress is immense. The rate of data generated each year globally is roughly of the order 10^18 bits. All of this data could be represented by a quantum computer possessing just 60 qubits, highlighting how revolutionary quantum computers will be for science. This article has attempted to explain the fundamental similarities these two fields exhibit and has given examples that demonstrate the collaboration that has occurred, and will continue to occur, between the fields of machine learning and quantum computing. This is truly an exciting marriage of fields that is evolving and developing before our eyes.
More from AZoQuantum: Why Quantum Encrypt Satellites?
References and Further Reading
Sankar Das Sarma, Dong-Ling Deng, Lu-Ming Duan, “Machine learning meets quantum physics”, arXiv:1903.03516, [physics.pop-ph], 2019
J. Carrasquilla, R.G. Melko, “Machine learning phases of matter”, arXiv:1605.01735, [cond-mat.str-el], 2016
G. Carleo, M. Troyer, Science, Vol. 355, Pg 602-606, 2017
J. Biamonte, P Wittek, N, Pancotti, P. Rebentrost, N. Wiebe, S. Lloyd, “Quantum Machine Learning”, arXiv:1611.09347v2, [quant-ph], 2018
S. Lloyd, M. Mohseni, P. Rebentrost, “Quantum algorithms for supervised and unsupervised machine learning”, arXiv:1307.0411v2, [quant-ph], 2013
E. M. Stoudenmire, D.J. Schwab, “Supervised Learning With Quantum-Inspired Tensor Networks”, arXiv:1605.05775v2, [stat.ML], 2017
R. Orus, “Tensor networks for complex quantum systems”, Nat Rev Phys 1, 538–550, https://doi.org/10.1038/s42254-019-0086-7, 2019
Disclaimer: The views expressed here are those of the author expressed in their private capacity and do not necessarily represent the views of AZoM.com Limited T/A AZoNetwork the owner and operator of this website. This disclaimer forms part of the Terms and conditions of use of this website.