A team of researchers, led by Yumin Dong from Chongqing Normal University, has introduced an innovative approach for enhancing parametric quantum circuits. These circuits are a crucial element of variational quantum algorithms.
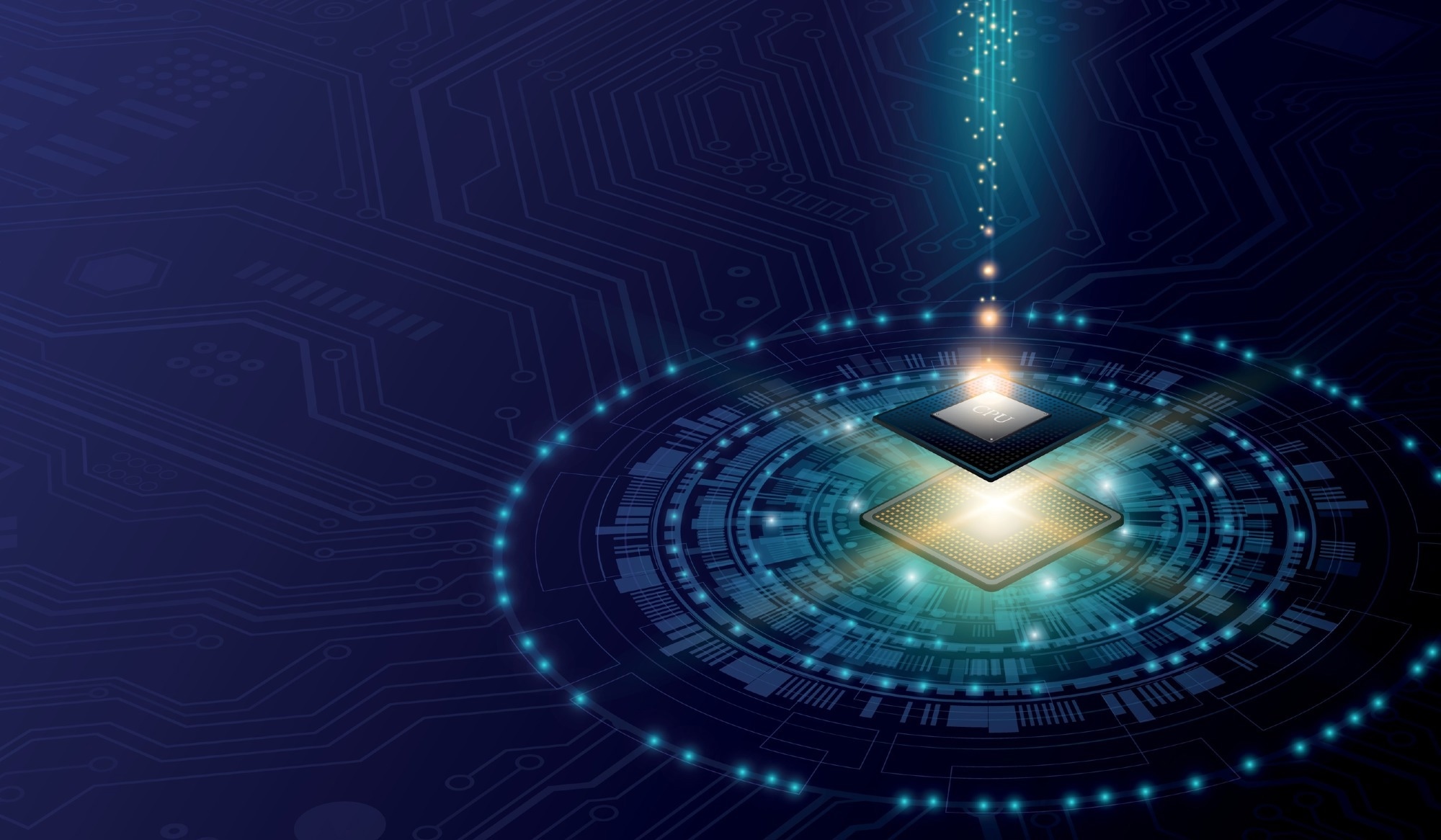
Image Credit: prawest/Shutterstock.com
Their method combines the effectiveness of a gradient-free natural evolutionary strategy with gradient descent techniques to address the challenging "barren plateau" problem often encountered in quantum computing. This breakthrough holds significant promise for the fields of quantum computing and machine learning.
Their study was reported in the journal Intelligent Computing on September 8th, 2023.
Variational quantum algorithms have significantly influenced the field of quantum computing, finding applications in quantum chemistry, combinatorial optimization, and machine learning tasks like information identification. Nevertheless, a persistent challenge in this domain has been the "barren plateau problem."
In classical optimization, gradient-based techniques rely on the gradient of the objective function, which acts like a slope to guide the optimization process. However, in the context of quantum computing, the barren plateau problem arises when quantum circuits exhibit an imperceptible gradient within the optimization problem's landscape. This makes traditional gradient-based optimization methods ineffective.
“Due to the nature of quantum computers, computing the gradient encounter many difficulties, such as numerous quantum computations and the accumulation of errors due to quantum noise and decoherence. These difficulties lead to the gradient vanishing problem.” Without a gradient to guide optimization, the situation is like being stuck on a barren plateau, making it challenging to find a solution.
Traditional optimization techniques have limitations when dealing with barren plateaus. Some previous ideas to address this issue, like quantum natural gradients and parameter initialization methods, also have limitations.
According to the researchers, “These strategies are expected to optimize quantum circuits in terms of the number of function evaluations and circuit size scaling. The gradient is estimated efficiently with constant evaluations, which remain unaffected by parameter count. Furthermore, these function evaluations are completely independent and can be executed in parallel. This means that these methods are suitable for high-dimensional problems.”
The research team introduced two distinct methods to tackle this challenge. The first method, referred to as NESSGD, combines a natural evolutionary strategy with stochastic gradient descent. The second method, known as NESAdaBelief, combines a natural evolutionary strategy with a variant of gradient descent. Both of these approaches are designed to optimize the parameters within parametric quantum circuits, particularly in the context of variational quantum algorithms.
They compared these methods with others in five classification tasks and found that the new methods performed better, showing their potential to improve quantum algorithm optimization.
To render their optimization approach feasible for quantum computing, the researchers investigated the adaptability of their evolutionary stochastic gradient descent variant with respect to the parameter shift rule.
This rule is a widely used technique for determining gradients within parametric quantum circuits. Their demonstration showcased the method's capability to conform to this rule, marking a significant step toward practical implementations on real quantum hardware.
The researchers said, “In the next step, we will consider combinatorial strategies for further improvement of training. Our goal is to identify a strategy that can stably mitigate or resolve the ‘barren plateau’ and provide theoretical support for the widespread use of variational quantum circuits.”
Journal Reference
Xie, J., et al. (2023) Natural Evolutionary Gradient Descent Strategy for Variational Quantum Algorithms. Intelligent Computing. doi.org/10.34133/icomputing.0042.