Theoretical physicists at RIKEN have achieved a significant improvement in the efficiency of a method for fixing errors in quantum computers. This advancement could be crucial for developing larger, more dependable quantum computers that utilize light. The study was published in Physical Review Letters.
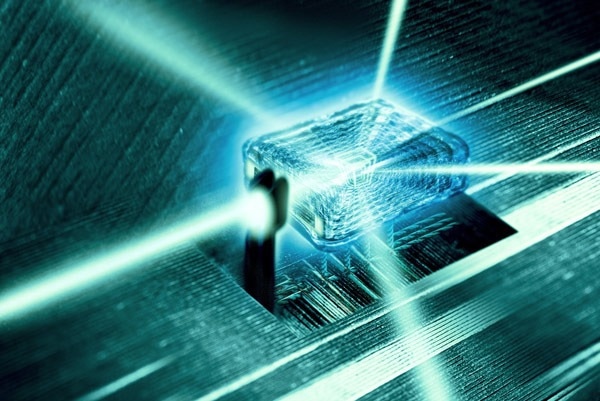
Quantum computers based on light stand to benefit from a technique that uses machine learning to enhance a method for fixing quantum errors. Image Credit: 2025 Richard Kail/Science Photo Library
Quantum computers are rapidly approaching realization and hold the potential to transform computing within the next 10 years.
Quantum computers have the potential to solve problems beyond the capabilities of today’s most powerful supercomputers.
Franco Nori, RIKEN Center for Quantum Computing (RQC)
However, significant challenges must be addressed before quantum computers can be effectively applied to real-world problems. A particularly crucial issue is ensuring the reliability of their calculations.
The reliability of quantum computers is constrained by their extreme sensitivity to the environment. Even minor environmental disturbances can corrupt quantum information.
Franco Nori, RIKEN Center for Quantum Computing (RQC)
Therefore, developing techniques to correct quantum errors is essential to unlock the full potential of quantum computers.
One promising approach is the Gottesman–Kitaev–Preskill (GKP) code. Introduced in 2001 by its creators, the GKP code offers a method to encode a qubit, the quantum counterpart of a classical bit, within a harmonic oscillator—a system analogous to a pendulum.
“It’s a promising candidate to realize quantum error correction without requiring a lot of hardware,” said Nori.
A significant challenge with GKP codes is their reliance on squeezed states (quantum states that are complex to create and manipulate). As a result, implementing GKP codes in real-world experiments, especially those using light, has proven difficult.
A team that includes Nori, along with Clemens Gneiting and Yexiong Zeng, both also affiliated with RQC, has developed a deep learning-based method to optimize GKP states. This optimization reduces the necessity for large-amplitude squeezed states while maintaining the code's robust error-correction capabilities.
By leveraging AI, our method fine-tunes the structure of GKP states, striking an optimal balance between resource efficiency and error resilience. Our optimized GKP codes outperform conventional ones, requiring only a fraction of the squeezed states while achieving superior error correction.
Yexiong Zeng, RIKEN Center for Quantum Computing (RQC)
The developed method proved to be surprisingly efficient, even surpassing the team's initial projections.
“We were pleasantly surprised. The neural network achieved a much more efficient encoding than we had initially expected,” said Zeng.
“By reducing resource demands and improving error resilience, our work accelerates the path toward scalable, fault-tolerant quantum computing, paving the way for significant advancements in science and technology,” said Gneiting.
Zeng stated that the team's next step is to expand the code to a system with multiple logical qubits.
Journal Reference:
Zeng, Y., et al. (2025) Neural-Network-Based Design of Approximate Gottesman-Kitaev-Preskill Code. Physical Review Letters. doi.org/10.1103/PhysRevLett.134.060601