A major obstacle facing quantum devices has been solved by a University of Oxford study that leveraged machine learning capabilities. The results show how to bridge the “reality gap,” or the discrepancy between expected and observed behavior from quantum devices, for the first time. Physical Review X has published the findings.
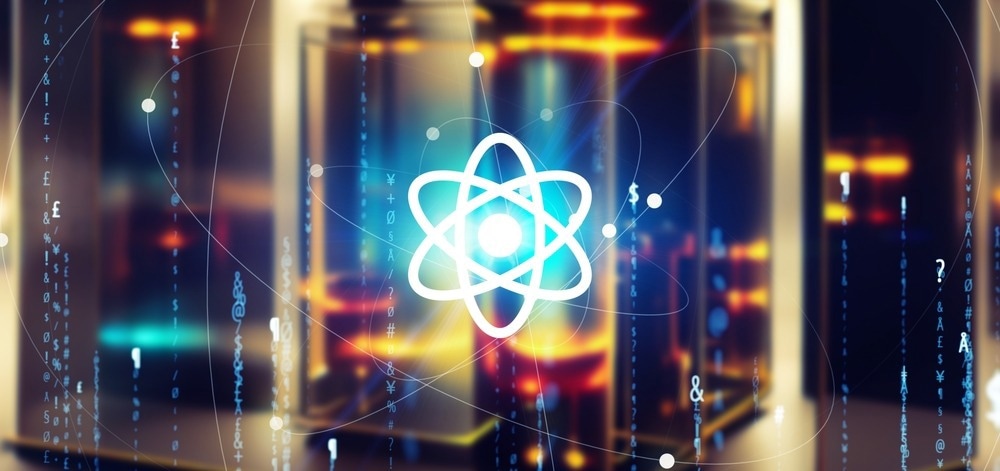
Image Credit: metamorworks/Shutterstock.com
Numerous applications, such as drug development, artificial intelligence, financial forecasting, and climate modeling, might be significantly improved by quantum computing. However, this will necessitate efficient methods for combining and scaling separate quantum bits (also known as qubits). Inherent variability, which occurs when even seemingly similar units display distinct behaviors, is a significant obstacle to this.
It is assumed that nanoscale flaws in the materials utilized to create quantum devices are the source of functional variability. This internal disorder cannot be represented in simulations since these cannot be measured directly, which accounts for the discrepancy between expected and observed results.
The study team addressed this by indirectly inferring certain disease traits through the use of a “physics-informed” machine learning technique. This was predicated on how the device’s intrinsic instability impacted the electron flow.
As an analogy, when we play “crazy golf” the ball may enter a tunnel and exit with a speed or direction that doesn’t match our predictions. But with a few more shots, a crazy golf simulator, and some machine learning, we might get better at predicting the ball’s movements and narrow the reality gap.
Natalia Ares, Study Lead Researcher and Associate Professor, Department of Engineering Science, University of Oxford
One quantum dot device was used as a test subject, and the researchers recorded the output current across it at various voltage settings. A simulation was run using the data to determine the difference between the measured current and the theoretical current in the absence of an internal disturbance.
The simulation was forced to discover an internal disorder arrangement that could account for the results at all voltage levels by monitoring the current at numerous distinct voltage settings. Deep learning was combined with statistical and mathematical techniques in this method.
Ares added, “In the crazy golf analogy, it would be equivalent to placing a series of sensors along the tunnel, so that we could take measurements of the ball’s speed at different points. Although we still can’t see inside the tunnel, we can use the data to inform better predictions of how the ball will behave when we take the shot.”
The novel model not only identified appropriate internal disorder profiles to explain the observed current levels, but it also demonstrated the ability to precisely forecast the voltage settings necessary for particular device operating regimes.
Most importantly, the model offers a fresh way to measure the differences in variability between quantum devices. This could make it possible to predict device performance more precisely and aid in the development of ideal materials for quantum devices. It could guide compensatory strategies to lessen the undesirable consequences of material flaws in quantum devices.
Similar to how we cannot observe black holes directly but we infer their presence from their effect on surrounding matter, we have used simple measurements as a proxy for the internal variability of nanoscale quantum devices. Although the real device still has greater complexity than the model can capture, our study has demonstrated the utility of using physics-aware machine learning to narrow the reality gap.
David Craig, Study Co-Author and PhD Student, Department of Materials, University of Oxford
Journal Reference
Craig, D. L., et. al. (2023) Bridging the Reality Gap in Quantum Devices with Physics-Aware Machine Learning. Physical Review X. doi:10.1103/PhysRevX.14.011001